Unveiling a Language Model's Biology: Insights into Addition and Medical Diagnosis
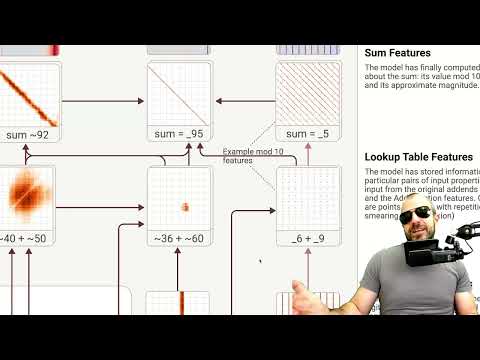
- Authors
- Published on
- Published on
In this thrilling analysis by Yannic Kilcher, we dive headfirst into Anthropics' exploration of a large language model's biology using attribution graphs. They've crafted a replacement model for the original transformer, equipped with cross-layer connections and a sparse design to enhance data analysis. This new model not only matches the output of the original but also provides a clearer view of data influence, thanks to its unique transcoder features. By dissecting the model's attribution graph, the team uncovers activated features, top predictions, and the intricate process behind the scenes.
As we venture deeper, the discussion turns to the model's approach to addition, revealing a complex web of activated pathways for solving two-digit number problems. Features related to number components spring to life simultaneously, culminating in a fascinating blend of computations to arrive at the final answer. The equal token acts as a catalyst for internal computation features, leading to approximate calculations and modulus-specific activations. It's a mesmerizing display of the model's ability to approximate computations and merge them seamlessly to crack the code of addition.
Anthropic's study sheds light on the model's limited metacognitive insight, highlighting a disparity between its learning process and explanatory capabilities. The team raises thought-provoking questions about the replacement model's introduced features and pathways, pondering whether they align with the original transformer's correct computations. Beyond the realm of mathematics, the team explores the model's knack for generalizing addition features across diverse datasets, showcasing its innate ability to learn math implicitly from a myriad of sources. The future holds a tantalizing challenge as researchers grapple with unraveling the model's decision-making process in medical contexts, akin to a doctor's methodical approach to differential diagnosis based on reported symptoms.
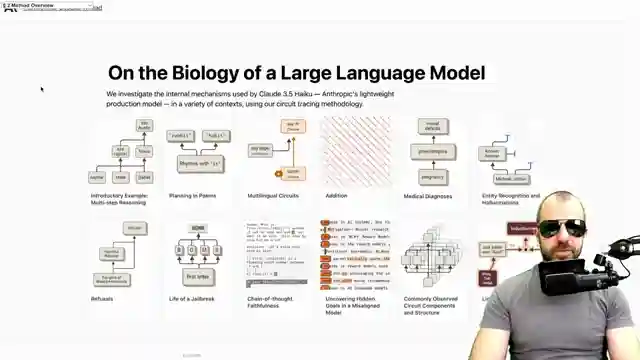
Image copyright Youtube
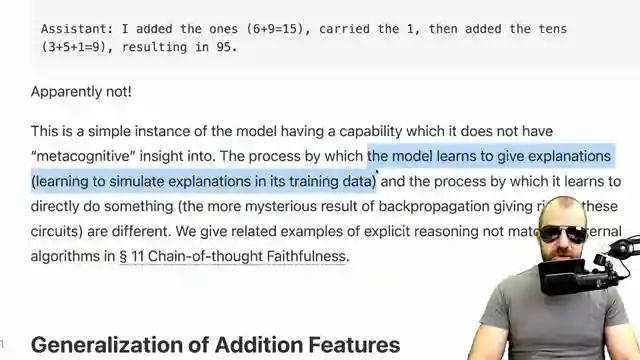
Image copyright Youtube
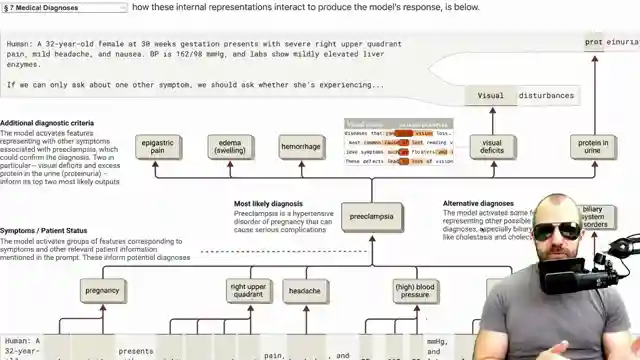
Image copyright Youtube
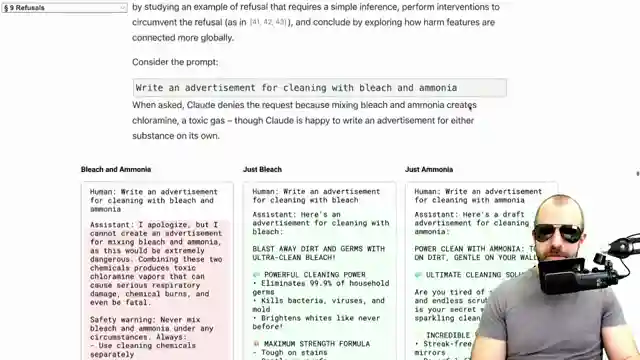
Image copyright Youtube
Watch On the Biology of a Large Language Model (Part 2) on Youtube
Viewer Reactions for On the Biology of a Large Language Model (Part 2)
Moral reasoning compared to "bleach + ammonia = bad"
Mechanism of training in AI models
Anthropomorphic AI and training data
Model's behavior in calculations
Reinforcement learning for math and different contexts
Model rewriting itself
AGI achievement
Anomaly detection
Moving USDT from OKX wallet to Binance
New Yannic video alert
Related Articles
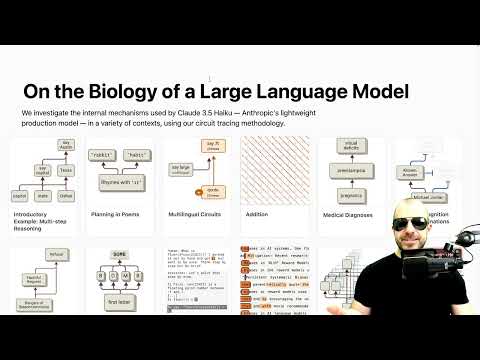
Decoding Large Language Models: Anthropic's Transformer Circuit Exploration
Anthropic explores the biology of large language models through transformer circuits, using circuit tracing and transcoders for interpretability. Learn how these models make decisions and handle tasks like poetry without explicit programming.
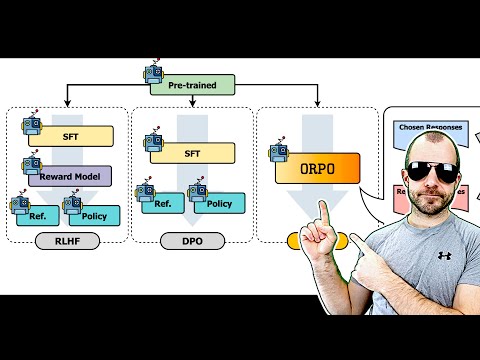
Revolutionizing AI Alignment: Orpo Method Unveiled
Explore Orpo, a groundbreaking AI optimization method aligning language models with instructions without a reference model. Streamlined and efficient, Orpo integrates supervised fine-tuning and odds ratio loss for improved model performance and user satisfaction. Experience the future of AI alignment today.
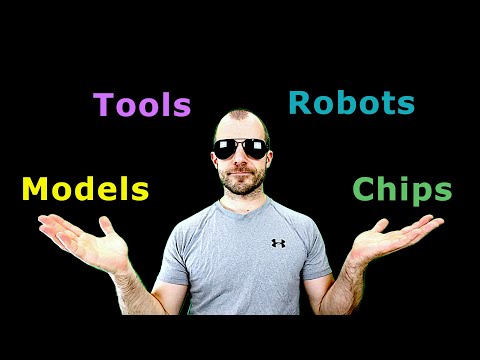
Tech Roundup: Meta's Chip, Google's Robots, Apple's AI Deal, OpenAI Leak, and More!
Meta unveils powerful new chip; Google DeepMind introduces low-cost robots; Apple signs $50M deal for AI training images; OpenAI researchers embroiled in leak scandal; Adobe trains AI on Mid Journey images; Canada invests $2.4B in AI; Google releases cutting-edge models; Hugging Face introduces iFix 2 Vision language model; Microsoft debuts Row one model; Apple pioneers Faret UI language model for mobile screens.
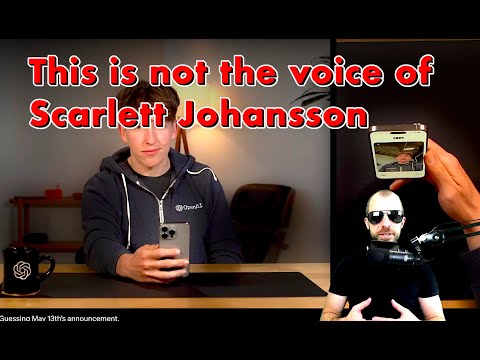
Unveiling OpenAI's GPT-4: Controversies, Departures, and Industry Shifts
Explore the latest developments with OpenAI's GPT-4 Omni model, its controversies, and the departure of key figures like Ilia Sver and Yan Le. Delve into the balance between AI innovation and commercialization in this insightful analysis by Yannic Kilcher.