Decoding Large Language Models: Anthropic's Transformer Circuit Exploration
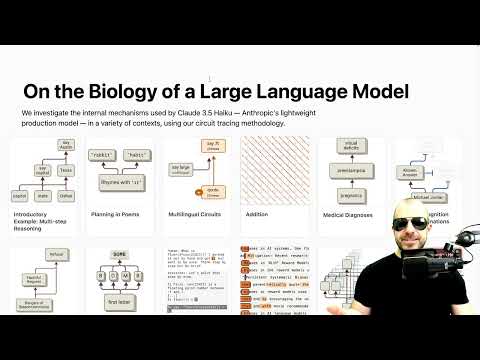
- Authors
- Published on
- Published on
In this riveting exploration of large language models, Anthropic takes us on a thrilling journey through the inner workings of transformer circuits. Forget about traditional programming - these models can compose poetry and tackle multilingual tasks without explicit instructions. Anthropic employs circuit tracing to dissect the features and decision-making processes of these models, shedding light on their mysterious capabilities. By using replacement models known as transcoders, they aim to make the complex workings of transformers more interpretable.
The transcoders, with their emphasis on sparsity and linear feature contributions, offer a clearer understanding of how information flows through the model. However, the road to interpretability is not without its challenges. Training these transcoders is a Herculean task, demanding significant computational resources and potentially sacrificing performance. The burning question remains - do these transcoders faithfully represent the behaviors of transformers, or do they lead us astray with misleading conclusions?
Through intervention experiments and attribution graphs, Anthropic strives to uncover the intricate dance between model features, input context, and desired output tokens. Features like "digital" and "DAG" come to life based on the context provided, showcasing the model's ability to navigate linguistic and semantic landscapes. By dissecting the model's decision-making processes, Anthropic offers a glimpse into the fascinating world of large language models and the biological problem of understanding their internal mechanisms.
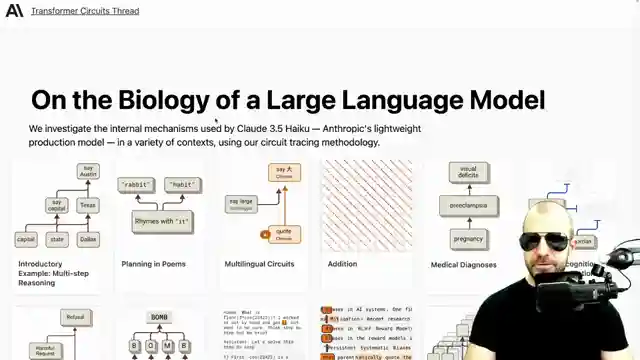
Image copyright Youtube
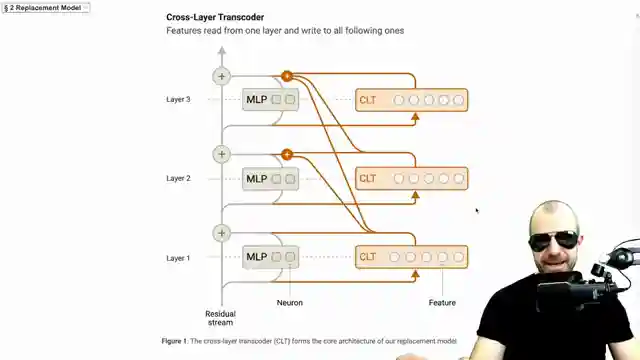
Image copyright Youtube
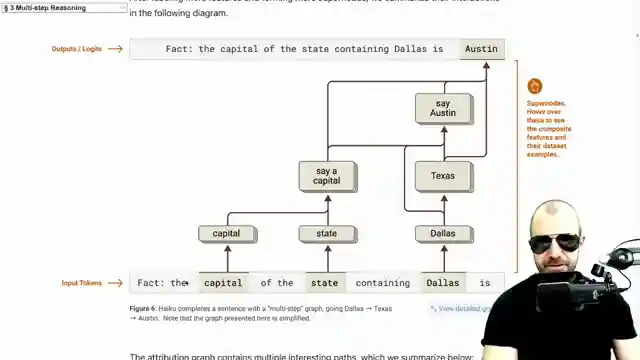
Image copyright Youtube
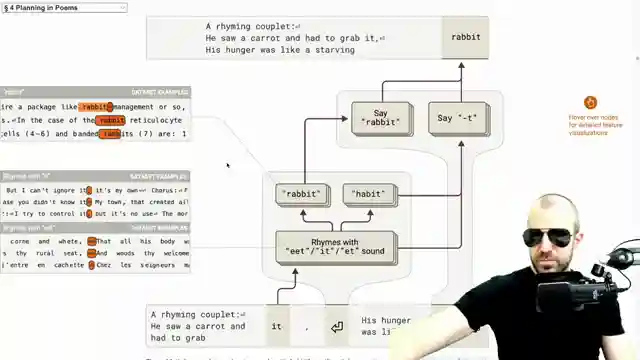
Image copyright Youtube
Watch On the Biology of a Large Language Model (Part 1) on Youtube
Viewer Reactions for On the Biology of a Large Language Model (Part 1)
Excitement for a new Yannic video
Discussion on the "On the "field" of an LLM" scheme
Appreciation for the paper being discussed
Questioning the intervention on the replacement model and its implications
Suggestions for features corresponding to concepts in multiple languages
Yannic Kilcher for president
Interest in the concept of a minimal universal language
Discord link expiration
Technical discussion on encoder tokenization stage
AI model recommendation of Yannic
Related Articles
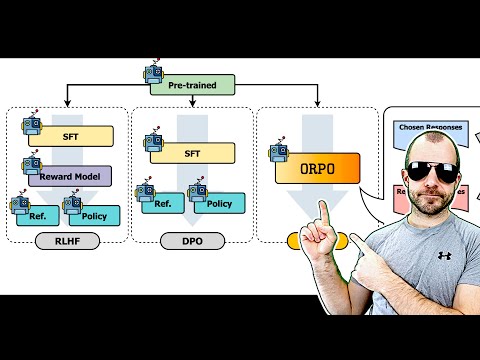
Revolutionizing AI Alignment: Orpo Method Unveiled
Explore Orpo, a groundbreaking AI optimization method aligning language models with instructions without a reference model. Streamlined and efficient, Orpo integrates supervised fine-tuning and odds ratio loss for improved model performance and user satisfaction. Experience the future of AI alignment today.
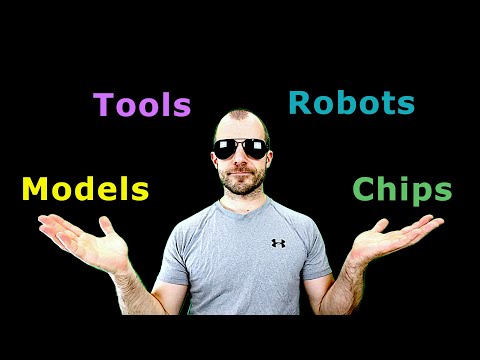
Tech Roundup: Meta's Chip, Google's Robots, Apple's AI Deal, OpenAI Leak, and More!
Meta unveils powerful new chip; Google DeepMind introduces low-cost robots; Apple signs $50M deal for AI training images; OpenAI researchers embroiled in leak scandal; Adobe trains AI on Mid Journey images; Canada invests $2.4B in AI; Google releases cutting-edge models; Hugging Face introduces iFix 2 Vision language model; Microsoft debuts Row one model; Apple pioneers Faret UI language model for mobile screens.
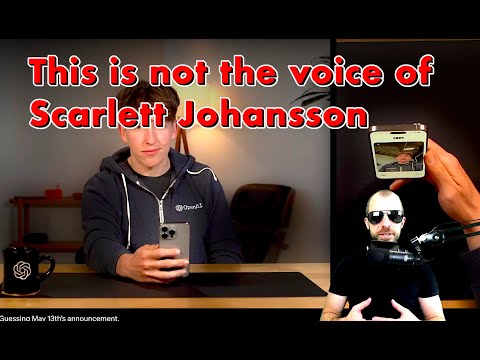
Unveiling OpenAI's GPT-4: Controversies, Departures, and Industry Shifts
Explore the latest developments with OpenAI's GPT-4 Omni model, its controversies, and the departure of key figures like Ilia Sver and Yan Le. Delve into the balance between AI innovation and commercialization in this insightful analysis by Yannic Kilcher.
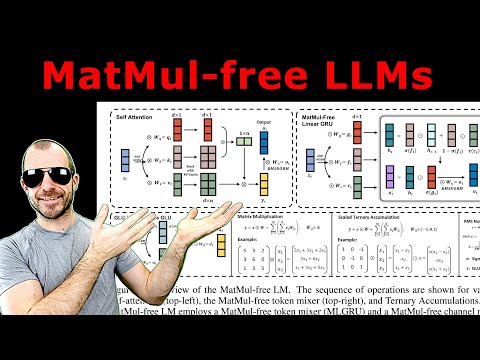
Revolutionizing Language Modeling: Efficient Tary Operations Unveiled
Explore how researchers from UC Santa Cruz, UC Davis, and Loxy Tech are revolutionizing language modeling by replacing matrix multiplications with efficient tary operations. Discover the potential efficiency gains and challenges faced in this cutting-edge approach.