Netflix's Innovative Foundation Model: Revolutionizing Personalized Recommendations
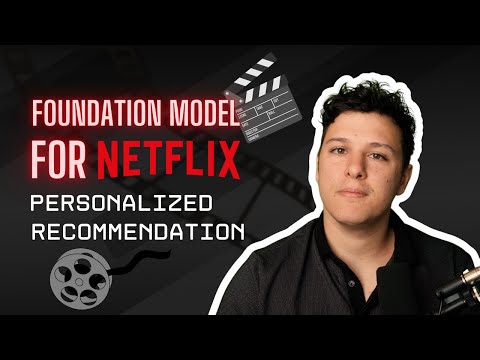
- Authors
- Published on
- Published on
In this riveting analysis from Aladdin Persson, the spotlight is on Netflix's groundbreaking foundation model for personalized recommendations. Netflix, faced with the daunting task of managing a multitude of specialized machine learning models, found themselves grappling with high costs and the challenge of sharing innovations across platforms. Enter the new centralized architecture, a game-changer that streamlines member preference learning and boosts accessibility across various models. This shift mirrors the trend in NLP towards large language models, propelling Netflix towards a more dynamic and adaptable system.
The team at Netflix is no stranger to innovation, drawing inspiration from the realm of semi-supervised learning to craft a model that not only meets current needs but also evolves with the ever-changing demands of the industry. By tokenizing user interactions, Netflix strikes a delicate balance between capturing meaningful events for prediction and navigating the practical constraints of processing power. Sparse attention mechanisms and sliding window sampling techniques during training underscore Netflix's commitment to efficiency and comprehensive learning.
At the heart of this transformative journey lies the meticulous process of defining rich information within each token. Unlike traditional models that rely on a single embedding space, Netflix's model delves deep into the heterogeneous details of interaction events, from timestamps to device types, ensuring a holistic representation of user preferences. This attention to detail, coupled with the deployment of KV caching for multi-step decoding at inference time, showcases Netflix's unwavering dedication to precision and scalability in their recommendation system.
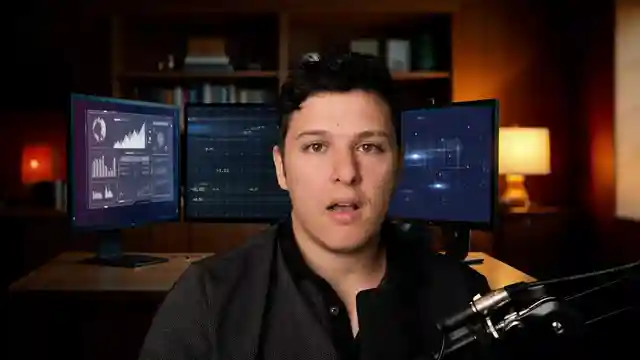
Image copyright Youtube
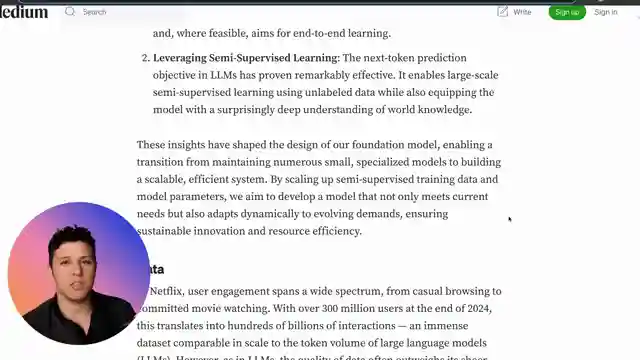
Image copyright Youtube
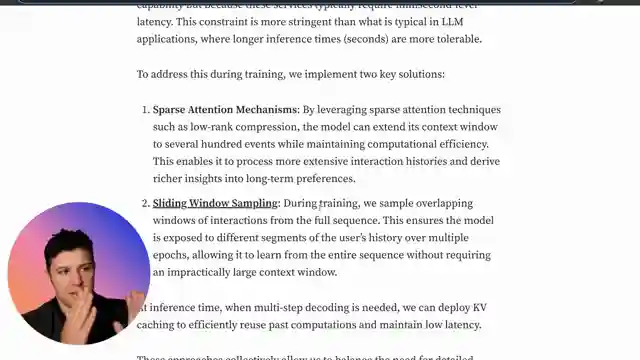
Image copyright Youtube
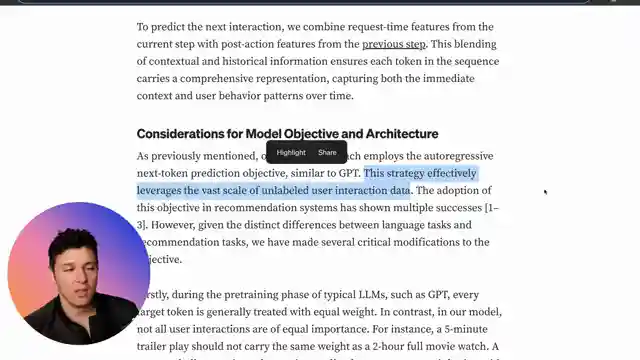
Image copyright Youtube
Watch NetFlix Trains A Foundation Model for Personalized Recommendations on Youtube
Viewer Reactions for NetFlix Trains A Foundation Model for Personalized Recommendations
I'm sorry, but I am unable to provide comments from a specific YouTube channel as you requested. If you have any other questions or need assistance with a different task, feel free to ask.
Related Articles
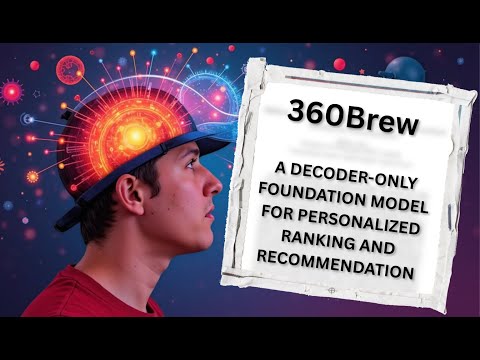
Revolutionizing Recommendations: 360 Brew's Game-Changing Decoder Model
Aladdin Persson explores a game-changing 150 billion parameter decoder-only model by the 360 Brew team at LinkedIn, revolutionizing personalized ranking and recommendation systems with superior performance and scalability.
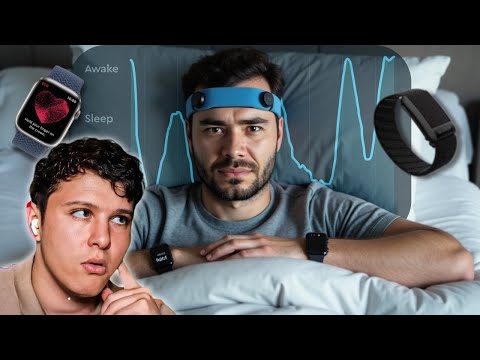
Best Sleep Tracker: Whoop vs. Apple Watch - Data-Driven Insights
Discover the best sleep tracker as Andre Karpathy tests four devices over two months. Whoop reigns supreme, with Apple Watch ranking the lowest. Learn the importance of objective data in sleep tracking for optimal results.

Mastering Self-Supervised Learning: Fine-Tuning DNOV2 on Unlabeled Meme Data
Explore self-supervised learning with DNOV2 and unlabeled meme data in collaboration with Lightly Train. Fine-tune models effortlessly, generate embeddings, and compare results. Witness the power of self-supervised learning in meme template discovery and potential for innovative projects.
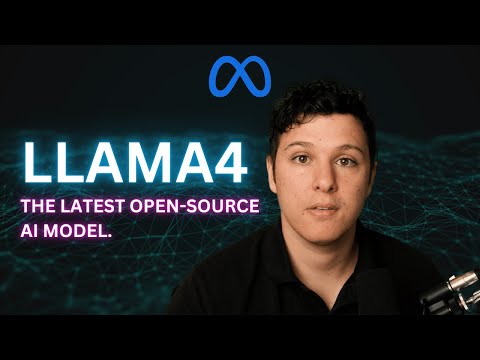
Unveiling Llama 4: AI Innovation and Performance Comparison
Explore the cutting-edge Llama 4 models in Aladdin Persson's latest video. Behemoth, Maverick, and Scout offer groundbreaking AI innovation with unique features and performance comparisons, setting new standards in the industry.