Enhancing Language Models: RAG vs CAG Techniques Explained
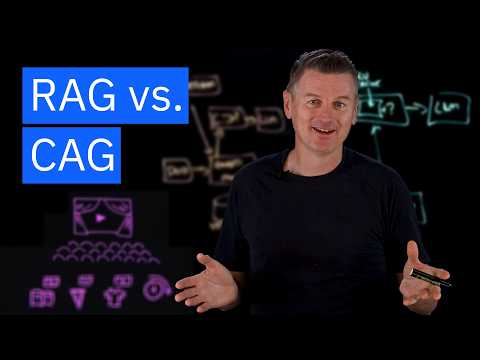
- Authors
- Published on
- Published on
In this riveting exploration by IBM Technology, we delve into the world of augmented generation techniques to supercharge those brainy language models. Picture this: Retrieval Augmented Generation (RAG), where the model goes on a thrilling quest through an external knowledge base to beef up its brainpower with relevant documents. On the flip side, we have Cache Augmented Generation (CAG), a method that stuffs all the knowledge into the model's brain at once. It's like cramming the entire library into your head before a quiz - intense stuff.
RAG, with its offline and online phases, is all about precision - like a sharpshooter aiming for accuracy tied to the retriever's performance. Meanwhile, CAG is the brute force approach, loading up on all potential info and daring the model to sift through it all. It's a battle of wits - finesse versus sheer horsepower. When it comes to speed, RAG adds an extra step in the workflow, while CAG blazes through with a single forward pass. It's like comparing a leisurely Sunday drive to a full-throttle race around the track.
Scaling up, RAG flexes its muscles, capable of handling massive datasets with ease, fetching only the juiciest bits. On the other hand, CAG hits a roadblock with its limited context size, forcing it to squeeze all that knowledge into a tight space. And let's not forget about data freshness - RAG's nimble footwork allows for quick updates, while CAG might stumble when the ground shifts beneath its feet. It's a showdown of adaptability and raw power, with each method bringing its own unique flair to the table. So, whether you're team RAG or team CAG, the choice is yours - pick your weapon and charge into battle with those language models!
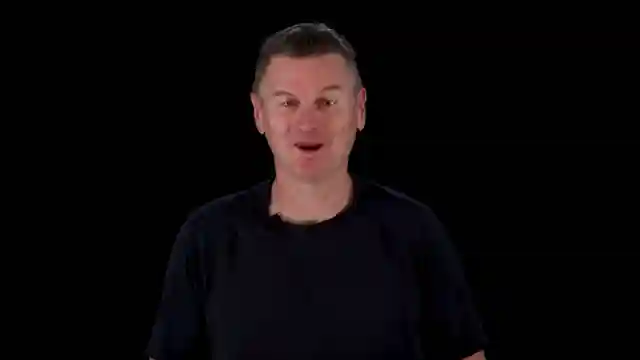
Image copyright Youtube
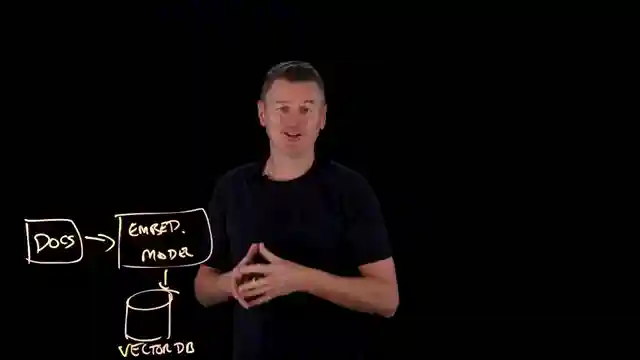
Image copyright Youtube
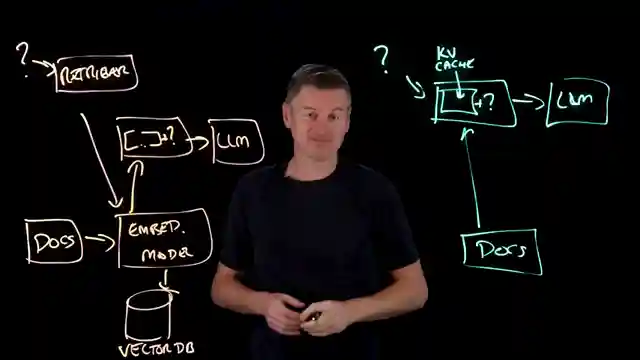
Image copyright Youtube
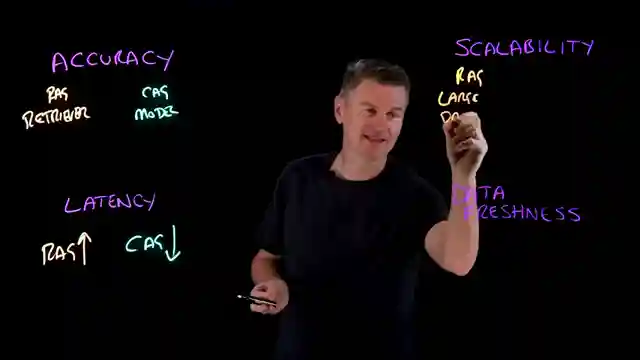
Image copyright Youtube
Watch RAG vs. CAG: Solving Knowledge Gaps in AI Models on Youtube
Viewer Reactions for RAG vs. CAG: Solving Knowledge Gaps in AI Models
Future job opportunities for Digital Transformation major
Implementation of CAG and its behavior compared to LLM
Use case limitations of CAG in handling large datasets
Concerns about cost and latency of CAG
Comparison between CAG and RAG
Effectiveness of LLM with smaller, relevant data
Concerns about CAG's context window size and cost
Mention of Voting Ensembles and IBM's position in AI
Specific scenario related to legal case transcripts and AI reasoning
Appreciation for the clear and informative content provided
Related Articles
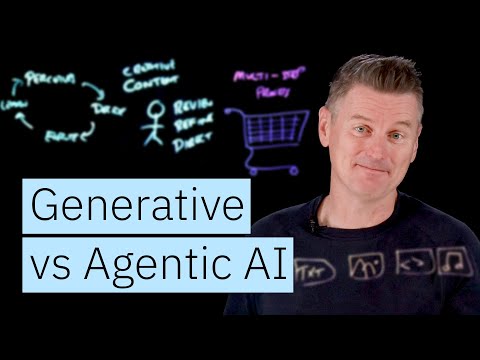
Decoding Generative and Agentic AI: Exploring the Future
IBM Technology explores generative AI and agentic AI differences. Generative AI reacts to prompts, while agentic AI is proactive. Both rely on large language models for tasks like content creation and organizing events. Future AI will blend generative and agentic approaches for optimal decision-making.
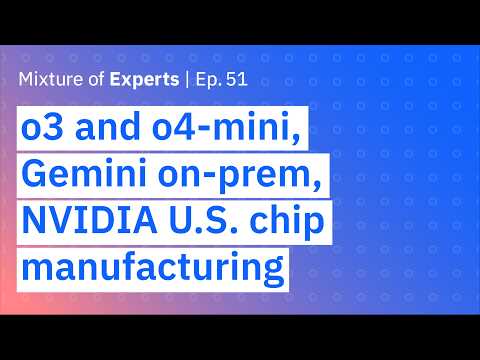
Exploring Advanced AI Models: o3, o4, o4-mini, GPT-4o, and GPT-4.5
Explore the latest AI models o3, o4, o4-mini, GPT-4o, and GPT-4.5 in a dynamic discussion featuring industry experts from IBM Technology. Gain insights into advancements, including improved personality, speed, and visual reasoning capabilities, shaping the future of artificial intelligence.
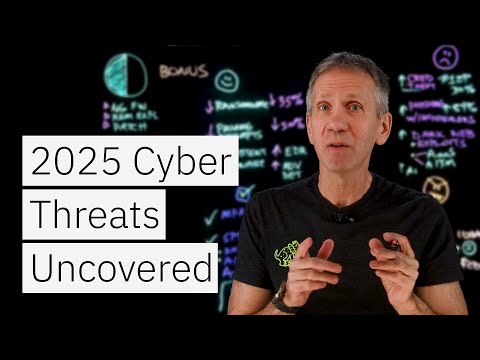
IBM X-Force Threat Intelligence Report: Cybersecurity Trends Unveiled
IBM Technology uncovers cybersecurity trends in the X-Force Threat Intelligence Index Report. From ransomware decreases to AI threats, learn how to protect against evolving cyber dangers.
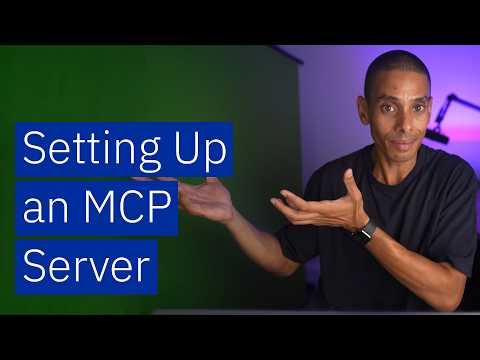
Mastering MCP Server Building: Streamlined Process and Compatibility
Learn how to build an MCP server using the Model Context Protocol from Anthropic. Discover the streamlined process, compatibility with LLMs, and observability features for tracking tool usage. Dive into server creation, testing, and integration into AI agents effortlessly.