Enhancing Language Models: RAG, Fine Tuning & Prompt Engineering
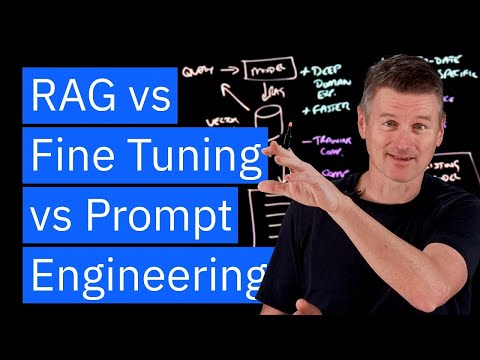
- Authors
- Published on
- Published on
In this riveting episode by IBM Technology, we delve into the fascinating world of enhancing large language models through innovative techniques like Retrieval Augmented Generation (RAG), fine tuning, and prompt engineering. It's like fine-tuning a high-performance car to extract every ounce of power. RAG involves scouring for fresh data, beefing up the prompt with newfound information, and crafting a response enriched with context. It's like giving your engine a shot of nitrous for an extra kick.
Fine tuning, on the other hand, is akin to customizing your ride with specialized parts to dominate the racetrack. By training a model on specific data sets, it gains profound domain expertise, tweaking its internal parameters for optimal performance. It's like transforming a regular sedan into a race-ready beast. And let's not forget prompt engineering, a sophisticated art form that fine-tunes the model's output without additional training or data retrieval. It's like adjusting your driving style to conquer any terrain effortlessly.
These methods, though distinct, can be seamlessly combined for maximum impact. Picture a legal AI system: RAG fetches specific cases and recent court decisions, prompt engineering ensures adherence to legal document formats, and fine tuning hones the model's grasp on firm-specific policies. It's like assembling a dream team of experts to tackle any challenge head-on. Each method offers its unique strengths - RAG expands knowledge, prompt engineering provides flexibility, and fine tuning cultivates deep domain expertise. It's all about choosing the right tool for the job and steering towards success in the ever-evolving landscape of language models.
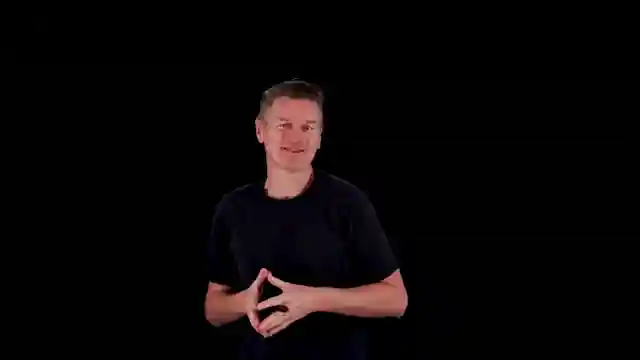
Image copyright Youtube
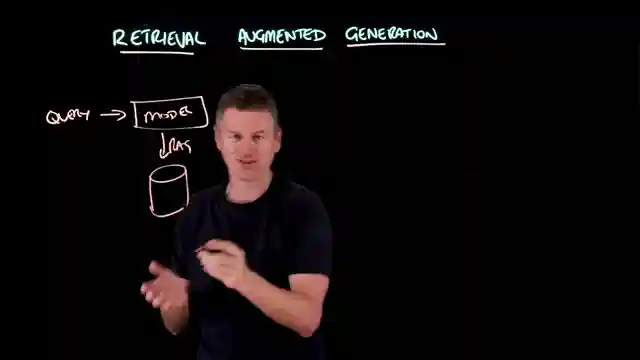
Image copyright Youtube
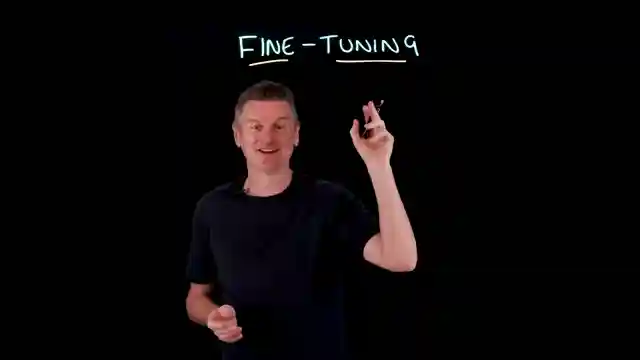
Image copyright Youtube
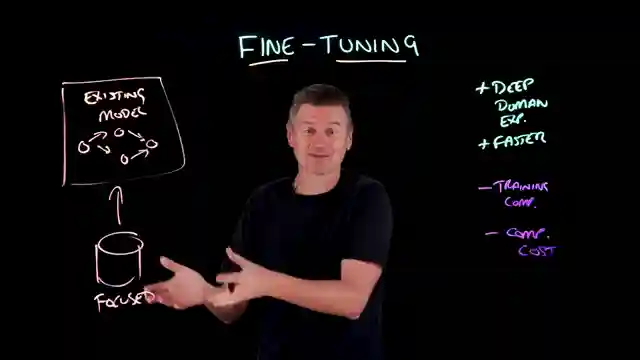
Image copyright Youtube
Watch RAG vs Fine-Tuning vs Prompt Engineering: Optimizing AI Models on Youtube
Viewer Reactions for RAG vs Fine-Tuning vs Prompt Engineering: Optimizing AI Models
Martin Keen is praised for his ability to explain complex topics clearly and in a fun way
LLMs like Gemini are mentioned for increasing awareness of Martin Keen's expertise in differentiating RAG, Fine-Tuning, and Prompt Engineering, along with AI model optimization strategies
Viewers are appreciative of the excellent video content and encourage more to be produced
Positive reactions such as "Great 👍" and "Sick" are expressed
Emojis like 😃 and 😊 are used to show appreciation and enjoyment of the content
Related Articles
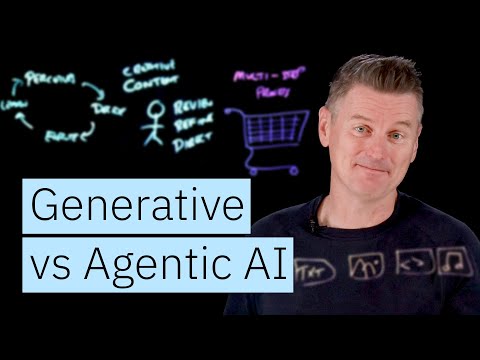
Decoding Generative and Agentic AI: Exploring the Future
IBM Technology explores generative AI and agentic AI differences. Generative AI reacts to prompts, while agentic AI is proactive. Both rely on large language models for tasks like content creation and organizing events. Future AI will blend generative and agentic approaches for optimal decision-making.
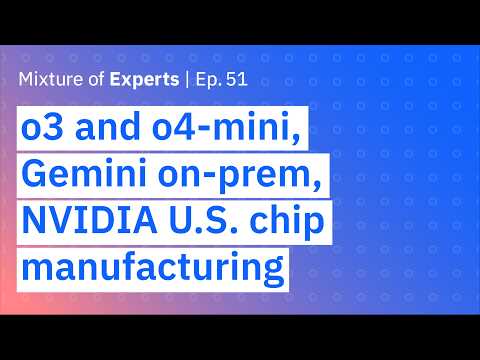
Exploring Advanced AI Models: o3, o4, o4-mini, GPT-4o, and GPT-4.5
Explore the latest AI models o3, o4, o4-mini, GPT-4o, and GPT-4.5 in a dynamic discussion featuring industry experts from IBM Technology. Gain insights into advancements, including improved personality, speed, and visual reasoning capabilities, shaping the future of artificial intelligence.
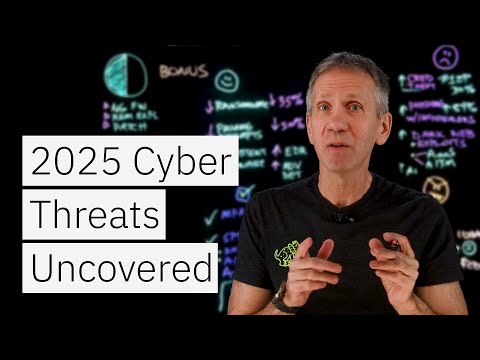
IBM X-Force Threat Intelligence Report: Cybersecurity Trends Unveiled
IBM Technology uncovers cybersecurity trends in the X-Force Threat Intelligence Index Report. From ransomware decreases to AI threats, learn how to protect against evolving cyber dangers.
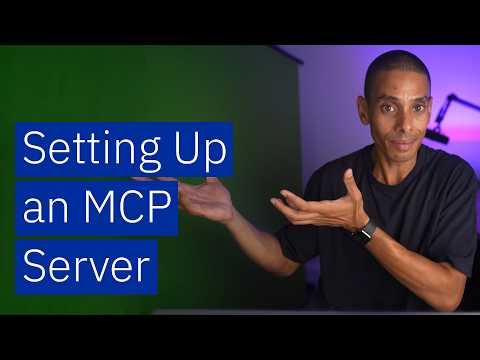
Mastering MCP Server Building: Streamlined Process and Compatibility
Learn how to build an MCP server using the Model Context Protocol from Anthropic. Discover the streamlined process, compatibility with LLMs, and observability features for tracking tool usage. Dive into server creation, testing, and integration into AI agents effortlessly.