Unleashing Agentic Rag: Revolutionizing AI Information Retrieval
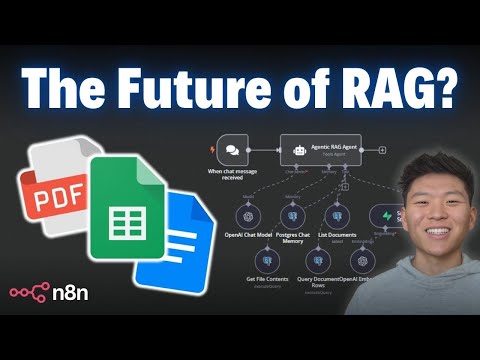
- Authors
- Published on
- Published on
In the thrilling world of AI development, the landscape of Rag, from KAG to Graph Rag, is shifting faster than a Bugatti Veyron on a racetrack. Enter Agentic Rag, a game-changer in the realm of Retrieval Augmented Generation. This cutting-edge technology allows agents to access databases, retrieve information, and generate precise answers with the finesse of a seasoned race car driver navigating a complex track. Traditional Rag may split documents into chunks and embed them for querying, but Agentic Rag brings a new level of reasoning to the table, considering various databases and schemas for more efficient and relevant results.
With the introduction of Gemini's million-context window, models can now read entire documents, providing a level of context akin to zooming out on a map to see the bigger picture. This means that when asking an AI to summarize a meeting or identify the week with the highest sales, Agentic Rag ensures accuracy by considering the full scope of information available. No more skimming the surface like a novice driver; this technology delves deep into the data, ensuring precision and reliability in every query.
Cole Mean's Agentic Rag template, a true marvel in the AI world, simplifies database setup in Superbase, making querying through documents and tabular data a breeze. This template, like a well-tuned engine, optimizes the process, allowing agents to navigate schemas and execute SQL queries with ease. By providing a structured framework for efficient data retrieval, this template empowers users to harness the full potential of Agentic Rag, much like a skilled driver mastering the intricacies of a high-performance vehicle on the track. So buckle up, embrace the thrill of AI innovation, and get ready to experience the exhilarating ride that is Agentic Rag.
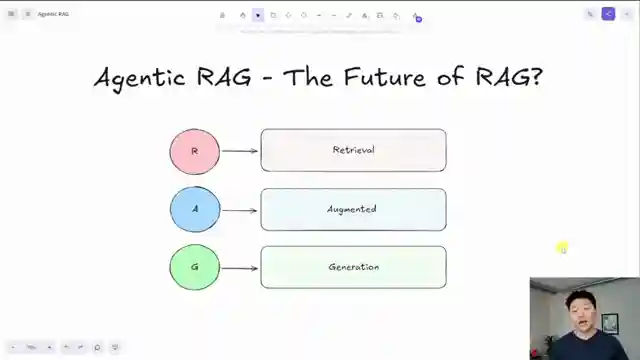
Image copyright Youtube
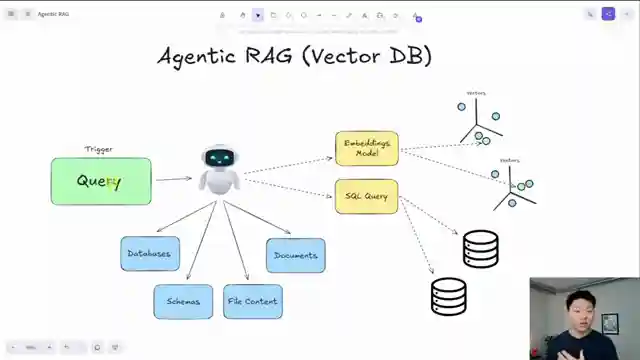
Image copyright Youtube
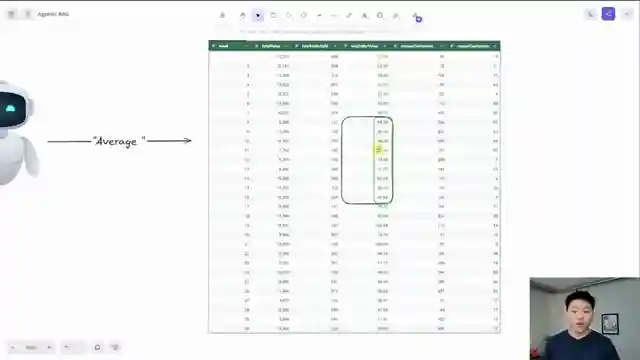
Image copyright Youtube
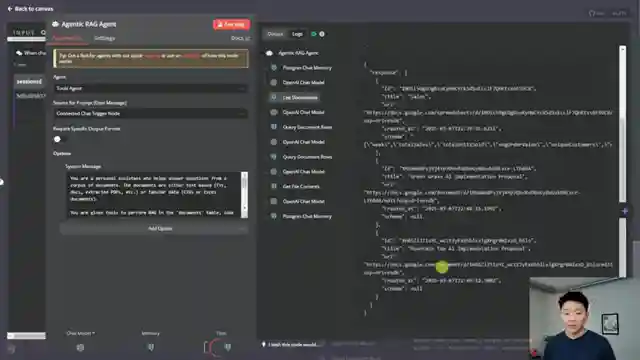
Image copyright Youtube
Watch Store All Data Types with Agentic RAG in n8n on Youtube
Viewer Reactions for Store All Data Types with Agentic RAG in n8n
Positive feedback on the video and explanation
Request for help on enhancing chatbot capabilities using data dictionary
Question about the limit of documents the RAG can handle
Query about selecting a directory in local n8n rag
Difficulty in understanding and using n8n for basic workflows
Request for advice on trading to make profit
Related Articles
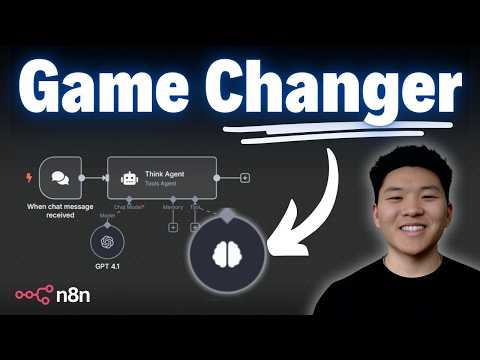
Ultimate Assistant: GPT 4.1 & Think Tool Showcase for AI Automation
Nate Herk showcases the Ultimate Assistant with GPT 4.1 and the Think Tool, demonstrating seamless task automation and problem-solving in AI workflows.
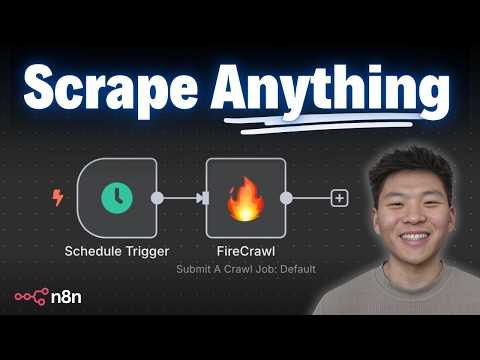
Master Website Data Extraction with FireCrawl and NN Integration
Explore FireCrawl's powerful website data extraction capabilities, from scraping to mapping. Learn how to extract specific content using prompts and automate the process with NN integration. Discover tips for efficient data extraction and overcoming challenges in this comprehensive guide.
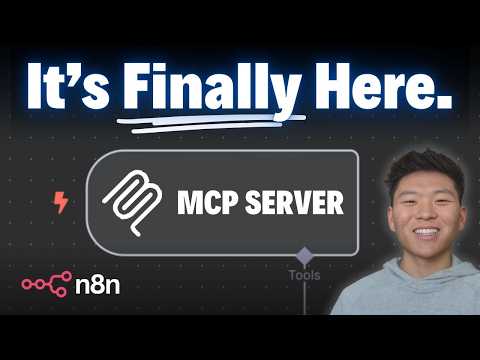
Naden's Native MCP Server Integration: Benefits, Limitations, and Demos
Naden's latest update introduces native integration for MCP servers, featuring the MCP server trigger and MCP client tool. Learn about the benefits, limitations, and practical demonstrations of this cutting-edge technology in AI automation.
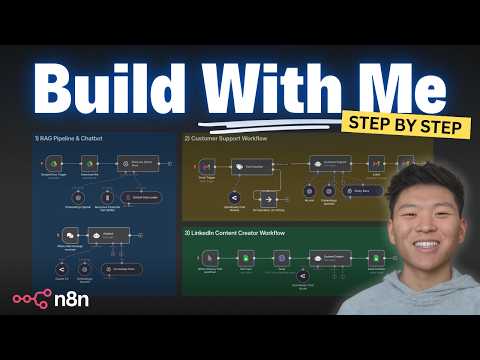
Master AI Workflows: Nate Herk's Guide to Rag Chatbots & Automation
Explore three exciting AI workflows on Nate Herk | AI Automation: rag Pipeline chatbot, customer support automation, and LinkedIn content creation. Learn to leverage Pinecone, Google Drive, NN AI agent, and Open Router for seamless automation. Master AI workflows and credential setup effortlessly.