Transforming LLM into Deep-Seek R1 Reasoner: Coding Tutorial
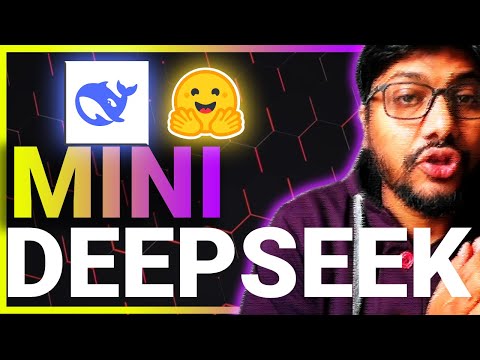
- Authors
- Published on
- Published on
In this coding tutorial from 1littlecoder, they embark on a thrilling journey to transform an LLM into a deep-seek R1 style Reasoner using the powerful GRPO technique. The team dives into the intricacies of the training process, emphasizing the importance of reward functions in shaping the model's behavior. From the simplicity of a basic question-answer format to the complex internal reasoning capabilities post-training, the evolution of the model is nothing short of mesmerizing.
With a nod to the original code's source from the internet, the team showcases a modified version that successfully runs on the Google Colab free notebook, showcasing the adaptability and resourcefulness required in the coding world. The discussion delves into the significance of choosing the right model size for optimal convergence, shedding light on the impact of model quality on training outcomes. As they navigate through setting up the model, defining training parameters, and fine-tuning learning rates, the audience is taken on a rollercoaster ride of coding expertise.
The tutorial doesn't shy away from the challenges faced during the experiment, including issues with batch size optimization and learning rate adjustments. Through meticulous monitoring of training metrics like XML reward count and KL Divergence, the team provides a transparent account of their coding escapades. Despite the model falling short of showcasing reasoning capabilities in this particular experiment, the tutorial serves as a testament to the unpredictable yet exhilarating nature of coding endeavors.
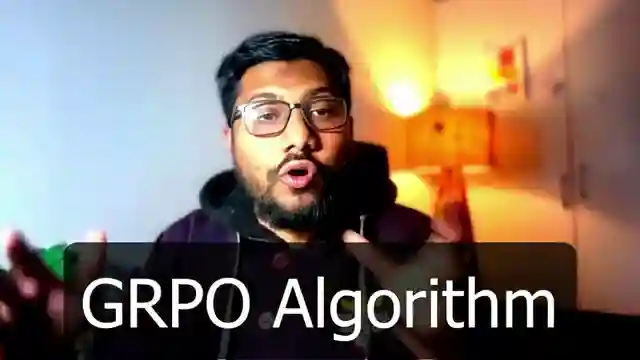
Image copyright Youtube
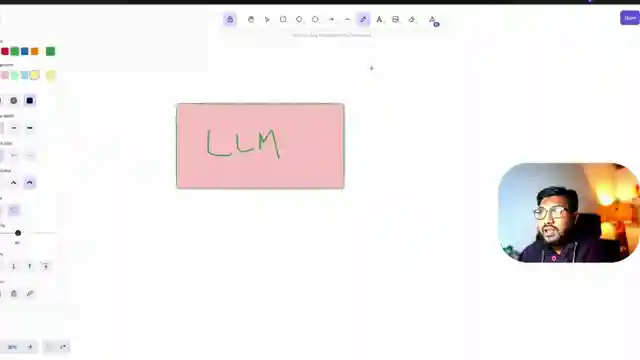
Image copyright Youtube
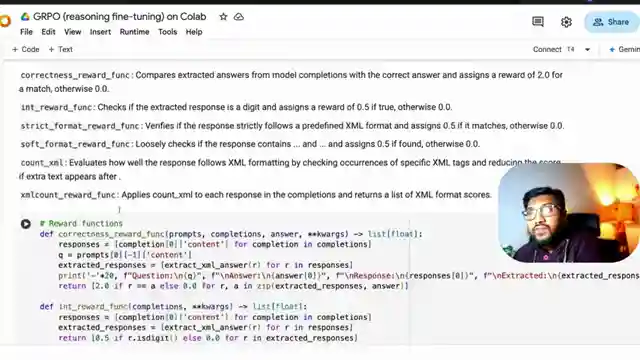
Image copyright Youtube
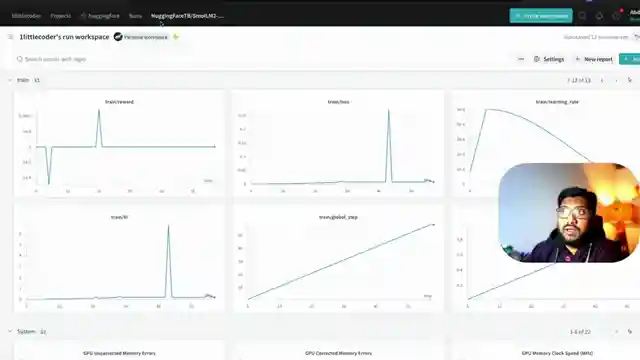
Image copyright Youtube
Watch Turn ANY LLM into a Mini Deepseek R1 💥Fine-Tuning with GRPO!!!💥 on Youtube
Viewer Reactions for Turn ANY LLM into a Mini Deepseek R1 💥Fine-Tuning with GRPO!!!💥
Positive feedback on the tutorial and appreciation for the value provided
Mention of a researcher finding 3B as the lower limit for reasoning
Comment on the unique way the number eight was written in the video
Interest in learning from mistakes shown in the tutorial
Request for another video if there are improvements in results
Intent to try out the tutorial
Mention of high VRAM usage for the tutorial
Excitement for future content related to phi-4 with grpo
Mention of the importance of giving credits when using code from others
Reference to the evolution of content creators in the field
Question about loading the tutorial into lm studio
Difficulty in getting the tutorial to work due to memory issues, specifically using a 3B Llama3 uncensored model
Note on errors in the Huggingface implementation
Mention of an upcoming OmniHuman 1 to look out for
Related Articles
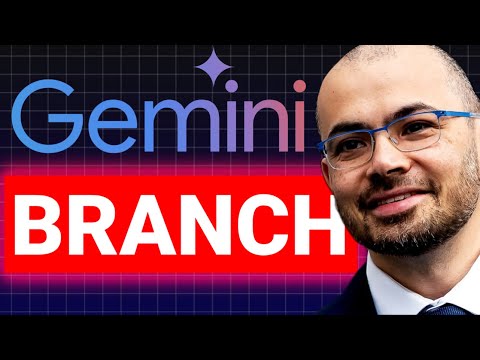
Unlock Productivity: Google AI Studio's Branching Feature Revealed
Discover the hidden Google AI studio feature called branching on 1littlecoder. This revolutionary tool allows users to create different conversation timelines, boosting productivity and enabling flexible communication. Branching is a game-changer for saving time and enhancing learning experiences.
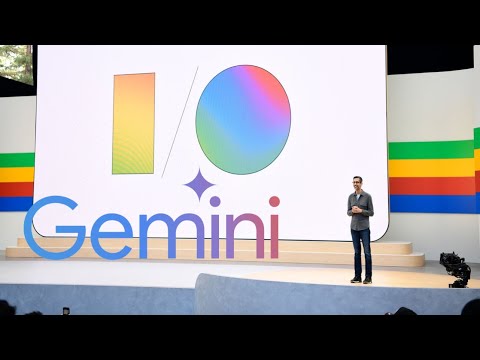
Revolutionizing AI: Gemini Model, Google Beam, and Real-Time Translation
1littlecoder unveils Gemini diffusion model, Google Beam video platform, and real-time speech translation in Google Meet. Exciting AI innovations ahead!
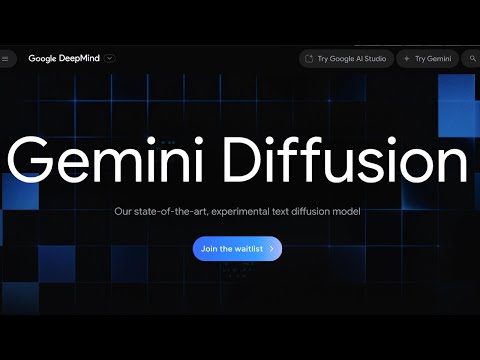
Unleashing Gemini: The Future of Text Generation
Google's Gemini diffusion model revolutionizes text generation with lightning-fast speed and precise accuracy. From creating games to solving math problems, Gemini showcases the future of large language models. Experience the power of Gemini for yourself and witness the next level of AI technology.
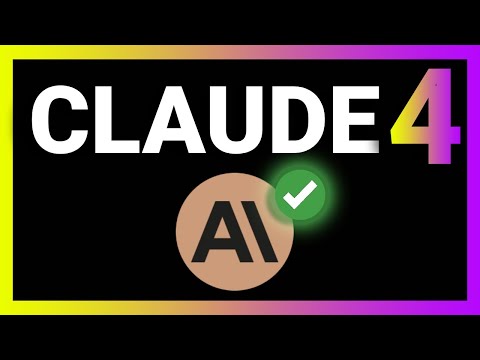
Anthropic Unleashes Claude 4: Opus and Sonnet Coding Models for Agentic Programming
Anthropic launches Claude 4 coding models, Opus and Sonnet, optimized for agentic coding. Sonnet leads in benchmarks, with Rakuten testing Opus for 7 hours. High cost, but high performance, attracting companies like GitHub and Manners.