Small Dockling: Precision OCR for Document Understanding
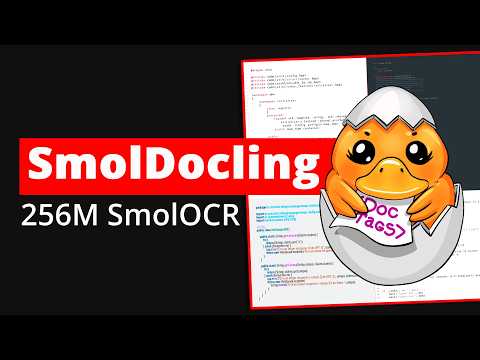
- Authors
- Published on
- Published on
In the realm of OCR models, Small Dockling from Hugging Face and IBM is like a compact sports car in a world of bulky SUVs. With just 256 million parameters, this little powerhouse can zoom through documents with precision, leaving competitors in the dust. While it may not be the biggest on the block, Small Dockling packs a punch by focusing not just on OCR but on the art of document conversion, a feat that sets it apart from the crowd. It claims to outshine rivals by a staggering 27 times, a bold statement that demands attention.
The Small Dockling project is not your run-of-the-mill OCR tool; it's a sophisticated extraction wizard capable of handling a variety of document formats with finesse. By combining a vision encoder and LM model, this OCR marvel delivers not only text recognition but also detailed location data in a sleek "dock tags" format, reminiscent of a high-tech blueprint. Its prowess extends to code recognition, formula extraction, and chart interpretation, making it a versatile contender in the OCR arena.
Available on Hugging Face, Small Dockling invites users to take it for a spin and experience its capabilities firsthand. While it may not be the ultimate OCR champion in every aspect, its compact size and focus on document conversion make it a compelling choice for those seeking tailored solutions. By offering fine-tuning options and script support, Hugging Face ensures that Small Dockling can be customized to excel in specific tasks, setting it apart as a nimble and adaptable tool in the world of OCR technology. Share your Small Dockling adventures in the comments and gear up for a thrilling ride through the realm of document understanding.
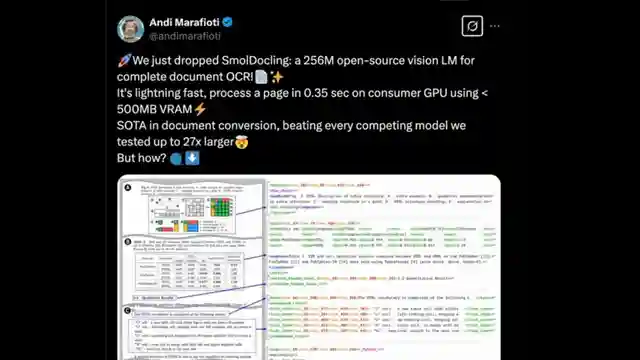
Image copyright Youtube
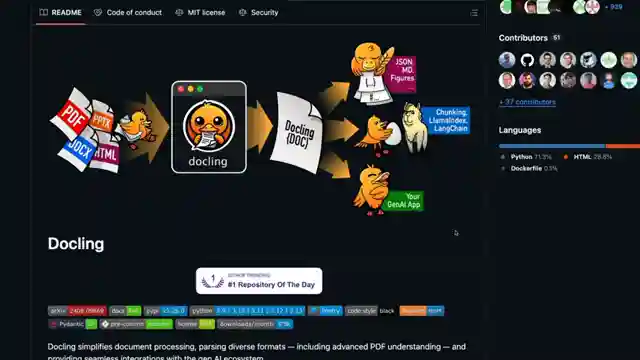
Image copyright Youtube
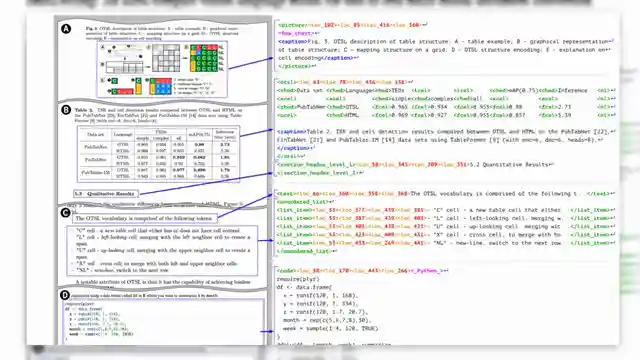
Image copyright Youtube
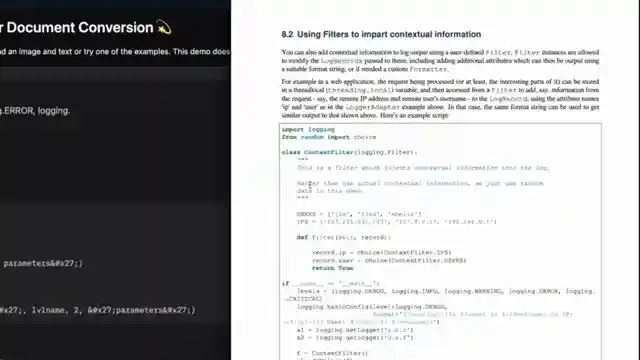
Image copyright Youtube
Watch SmolDocling - The SmolOCR Solution? on Youtube
Viewer Reactions for SmolDocling - The SmolOCR Solution?
SmolDocling is seen as a replacement for OCR, offering more than just text extraction
Docling is praised for its accuracy and speed compared to other models like Gemini and Mistral
Users are curious about the integration of Tesseract engine and its compatibility with Docling
Some users are interested in using Docling for specific tasks like RAG pipeline and transforming outputs into LaTeX
Questions about the model's performance with different languages, handwriting, and maximum resolution
Comparison requests between SmolDocling and Donut
Interest in using Docling for detecting specific elements like headlines or CTAs in static display ads
Suggestions for fine-tuning the model for specific use cases, such as handling shorthand
Some users express difficulty or dissatisfaction with the results obtained from using Docling
Speculation on the main purpose of the model being to publish a paper about it
Related Articles
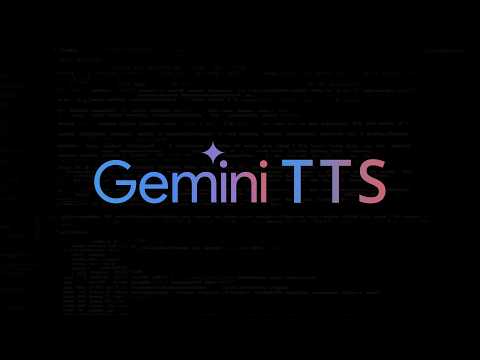
Unveiling Gemini 2.5 TTS: Mastering Single and Multi-Speaker Audio Generation
Discover the groundbreaking Gemini 2.5 TTS model unveiled at Google IO, offering single and multi-speaker text to speech capabilities. Control speech style, experiment with different voices, and craft engaging audio experiences with Gemini's native audio out feature.
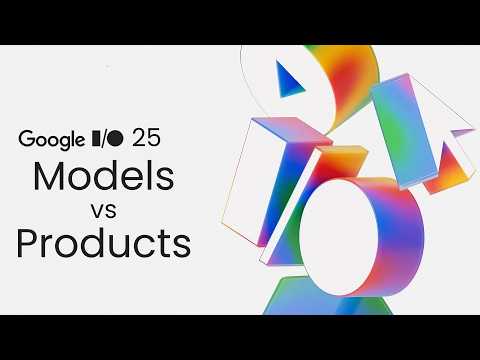
Google IO 2025: Innovations in Models and Content Creation
Google IO 2025 showcased continuous model releases, including 2.5 Flash and Gemini Diffusion. The event introduced Image Gen 4 and VO3 video models in the innovative product Flow, revolutionizing content creation and filmmaking. Gemini's integration of MCP and AI Studio refresh highlight Google's commitment to technological advancement and user empowerment.
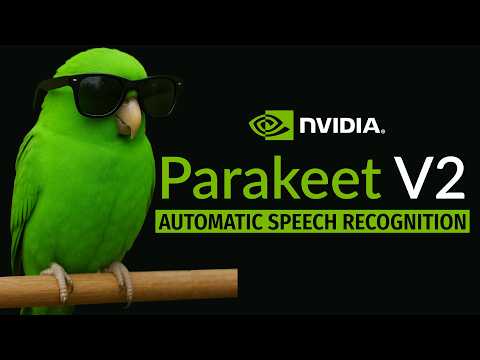
Nvidia Parakeet: Lightning-Fast English Transcriptions for Precise Audio-to-Text Conversion
Explore the latest in speech-to-text technology with Nvidia's Parakeet model. This compact powerhouse offers lightning-fast and accurate English transcriptions, perfect for quick and precise audio-to-text conversion. Available for commercial use on Hugging Face, Parakeet is a game-changer in the world of transcription.
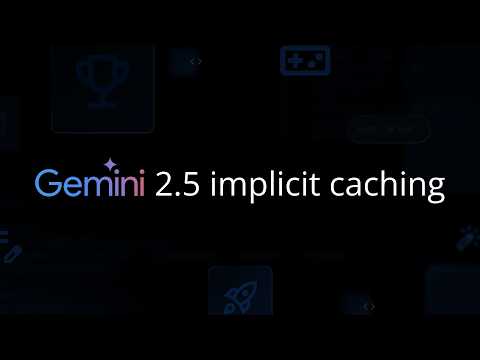
Optimizing AI Interactions: Gemini's Implicit Caching Guide
Gemini team introduces implicit caching, offering 75% token discount based on previous prompts. Learn how it optimizes AI interactions and saves costs effectively. Explore benefits, limitations, and future potential in this insightful guide.