Nvidia GTC 2025: Unveiling Llama Neotron Super 49b V1 and Model Advancements
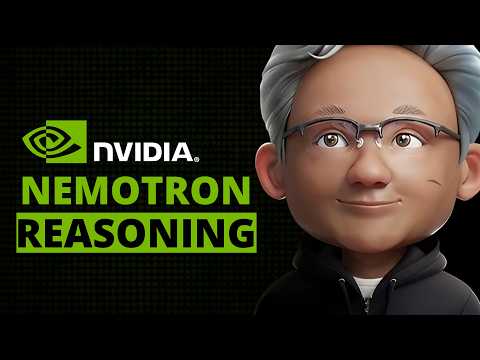
- Authors
- Published on
- Published on
In this latest Nvidia extravaganza at GTC 2025, Jensen H takes the stage to unveil the company's latest data center innovations. What used to be a developer-centric affair has now morphed into a spectacle aimed squarely at investors. The star of the show? Nvidia's reasoning models, promising a surge in tokens for a myriad of tasks. Enter the llama neotron models, building on the llama 3.1 and 3.3 foundations, with the new llama 3.3 neotron super 49b V1 stealing the spotlight. It sounds like a weapon from a sci-fi flick, but it's all about distilled power from the 70b model.
But why is Nvidia hitching its wagon to the llama series instead of blazing its own trail? With ample GPUs and a crack team of researchers, one would think they'd go solo. Instead, they're tinkering with meta ai's llama models, experimenting with post-training techniques and reinforcement learning. The release of the 49b and 8b models, along with a generous post-training dataset, signals Nvidia's foray into democratizing model training. The dataset, boasting millions of samples across various domains, is a goldmine for those venturing into reasoning model development.
The real test comes when users dive into Nvidia's API to put these models through their paces. The ability to toggle detailed thinking on and off adds a layer of intrigue to the experience. While the 49b model shines with its high-quality thinking reminiscent of Deep seek, the 8b model falls short of expectations. Nvidia's move is bold, but questions linger about the 8b model's viability compared to existing alternatives. As the community delves into these models and shares feedback, the debate over optimal model sizes for local versus cloud usage rages on. With links to code and demos provided, the stage is set for enthusiasts to explore Nvidia's latest offerings and push the boundaries of reasoning model capabilities.
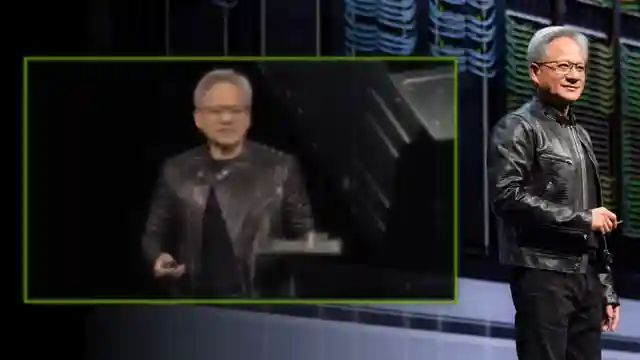
Image copyright Youtube
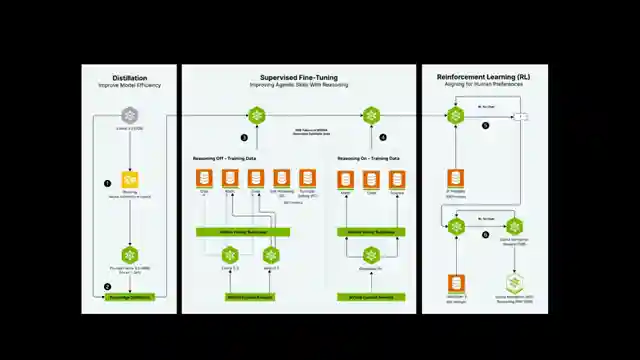
Image copyright Youtube
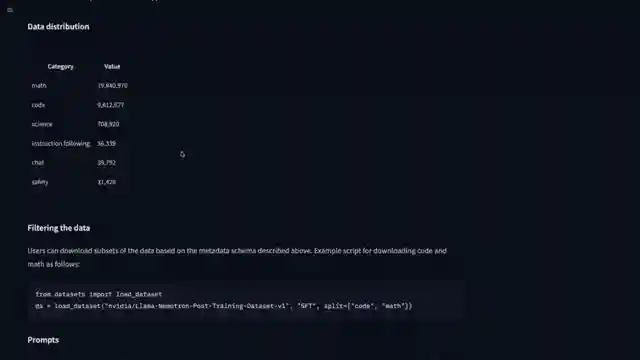
Image copyright Youtube
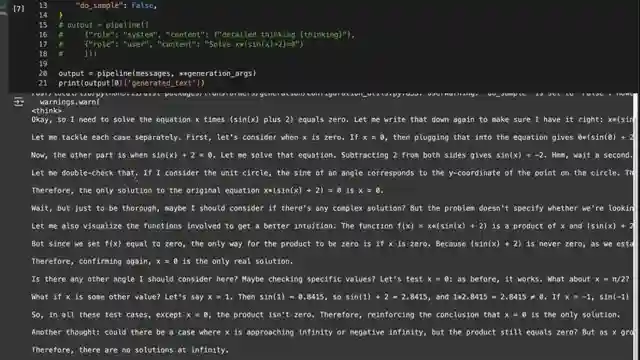
Image copyright Youtube
Watch NVIDIA's New Reasoning Models on Youtube
Viewer Reactions for NVIDIA's New Reasoning Models
Using techniques outside of base model building in Syria makes sense for selling semiconductors
Limited availability of 200 5090s at launch across all US Microcenters
Suggestions for Nvidia to use different base models like qwen instead of llama
Speculation on Nvidia completely changing the original Llama model
Questioning why Nvidia doesn't just inject "think" via chat template
Concerns about Nvidia training its own LLMs from scratch due to legal issues and copyright concerns
Excitement about using the technology on an rx 7900 xtx with ROCm
Criticism of Nvidia for selling excessive energy waste caused by inefficient design applications
Hope for Chinese companies to make Nvidia irrelevant in the AI industry
Related Articles
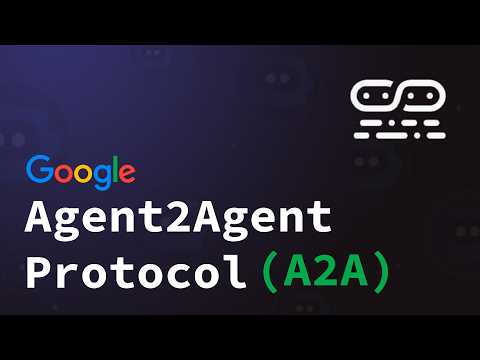
Exploring Google Cloud Next 2025: Unveiling the Agent-to-Agent Protocol
Sam Witteveen explores Google Cloud Next 2025's focus on agents, highlighting the new agent-to-agent protocol for seamless collaboration among digital entities. The blog discusses the protocol's features, potential impact, and the importance of feedback for further development.
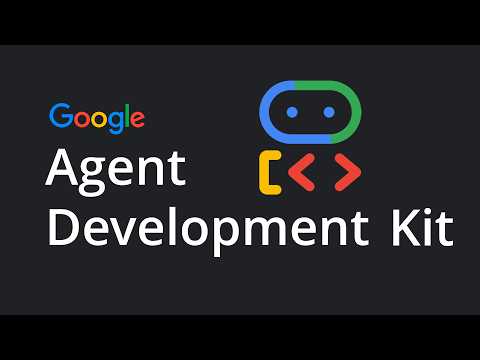
Google Cloud Next Unveils Agent Developer Kit: Python Integration & Model Support
Explore Google's cutting-edge Agent Developer Kit at Google Cloud Next, featuring a multi-agent architecture, Python integration, and support for Gemini and OpenAI models. Stay tuned for in-depth insights from Sam Witteveen on this innovative framework.
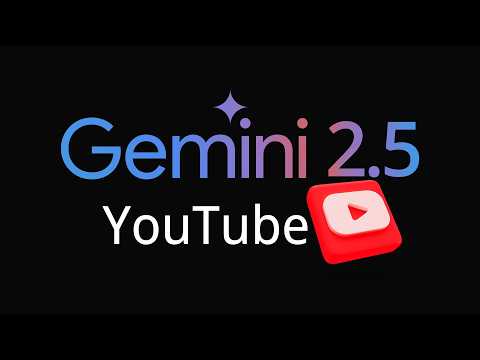
Mastering Audio and Video Transcription: Gemini 2.5 Pro Tips
Explore how the channel demonstrates using Gemini 2.5 Pro for audio transcription and delves into video transcription, focusing on YouTube content. Learn about uploading video files, Google's YouTube URL upload feature, and extracting code visually from videos for efficient content extraction.
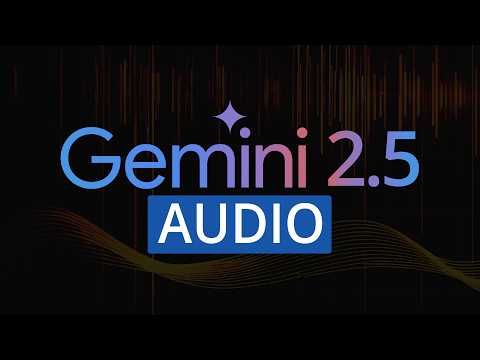
Unlocking Audio Excellence: Gemini 2.5 Transcription and Analysis
Explore the transformative power of Gemini 2.5 for audio tasks like transcription and diarization. Learn how this model generates 64,000 tokens, enabling 2 hours of audio transcripts. Witness the evolution of Gemini models and practical applications in audio analysis.